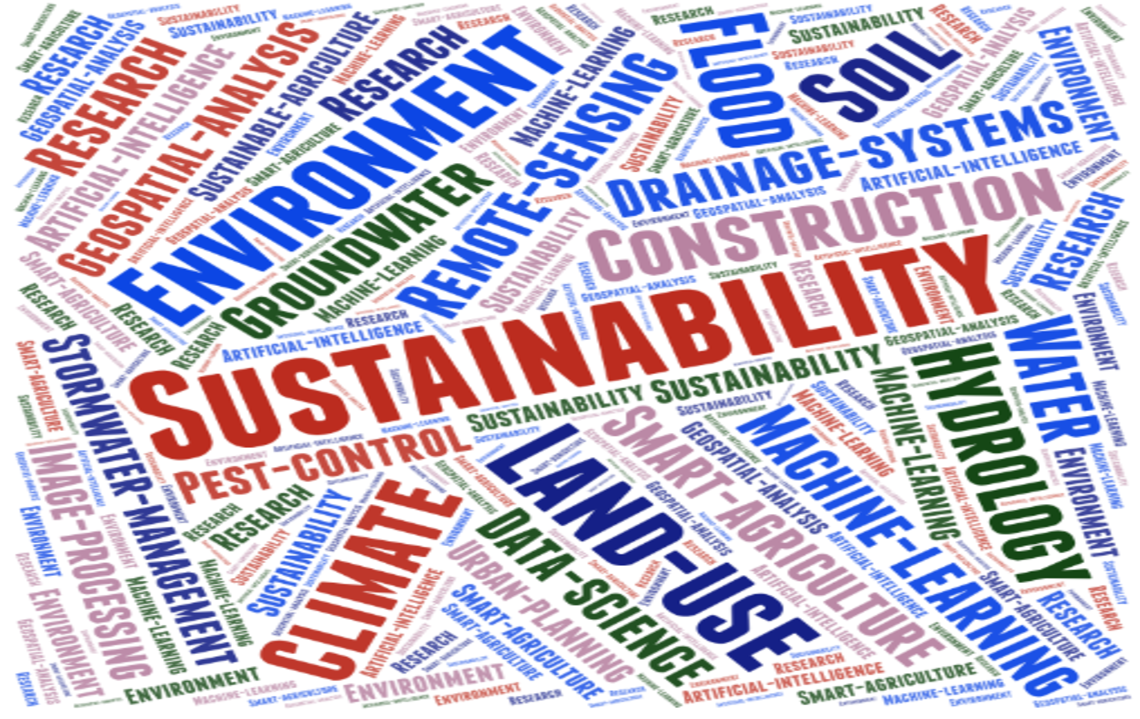
Research Focus
My key research focuses on sustainability in both agriculture and the environment, aiming to make meaningful contributions to global challenges.
My key research focuses on sustainability in both agriculture and the environment, aiming to make meaningful contributions to global challenges.
Title: Bayesian optimized multimodal deep hybrid learning approach for tomato leaf disease classification.
Journal: Scientific Reports (Nature Portfolio)
Designed a sustainable flood mitigation approach using machine leraning, remote sensing and hydrologic-hydraulic simulation. This research was recognised by the Ministry of Science and Technology, Bangladesh.
Ongoing research on energy systems using SciML.This is a collaboration work with the researchers from Columbia University in The City of New York and University of Science and Technology of China.
Ongoing research on object detection using advanced customized ViT framework.This is a collaboration work with the researchers from Sylhet Agricultural University.
Ongoing research on outage prediction using Hybrid DL.This is a collaboration work with the researchers from Cornell University and Columbia University in The City of New York.
A manuscript (collaboration work with Sylhet Agricultural University) has been submitted to the journal of Computers and Electronics in Agriculture on the study of climate change on rice production.
A practical application of Python in developing user-friendly software solutions, enabling efficient and accurate analysis of soil classes for professionals.
I have done several practical projects related to remote sensing and spatial analysis where I used the Google Earth Engine platform. Along with GEE, I regularly use other spatial tools like GIS and ArcGIS.
I use professional design programs, such as AutoCAD and SkechUp, for 2D and 3D drawing tasks.
Using physics-informed neural networks (PINNs), I was able to model the complex interactions that govern fluid behavior around rotating bodies.
I have modeled an interpretable machine learning approach for land cover classifications. This interpretability is crucial for the reliability of ML in practical cases.
My research interests lie at the intersection of machine learning, sustainable agriculture, and environmental management. I aim to explore innovative methodologies for addressing climate change and resource management.
Master’s Degree in Agricultural Construction and Environmental Engineering CGPA: 3.962/4 | Completion Year: 2024 Bachelor’s Degree in Agricultural Engineering and Technology CGPA: 3.846/4 | Completion Year: 2022
I employ wide range methodologies, including applied machine learning, hierarchical deep learning, explainable AI, geospatial analysis, hydrological simulation, remote sensing, image classification, spatio-temporal analysis, and time-series forecasting.
Proficient in Python, with extensive real-life experience in deep learning, supervised and unsupervised learning, statistical analysis, engineering design and modeling, and geospatial technologies including ArcGIS, QGIS, and Google Earth Engine.
Engage with emerging technologies such as AI, computer vision, and deep learning to enhance data-driven decision-making in sustainable agriculture and environmental management, fostering innovative solutions for global environmental challenges.
Active participation in research collaborations and academic networks to foster interdisciplinary approaches towards global agri-environmental issues. I am open to opportunities for Novel studies, as I aspire to contribute to impactful research that addresses global challenges.